China has witnessed the same degree of industrialisation in three decades as Europe did in two centuries – without the preconditions that are generally believed to be necessary for economic development, that is, effective legal systems or well-functioning financial institutions. Analysing unique administrative data on the universe of registered firms in the country, this article shows that community networks organised around the birth county paved the way for entrepreneurs to flourish.
China has witnessed the same degree of industrialisation in three decades as Europe did in two centuries (Summers 2007). This economic transformation began in the early 1980s with the establishment of township-village enterprises (TVEs) and accelerated with the entry of private firms in the 1990s. Starting with almost no private firms in 1990, there were 15 million registered private firms in 2014, accounting for over 90% of all registered firms and 60% of aggregate industrial production.
Figure 1. Distribution of firms, by type
a) Number of firms
b) Registered capital
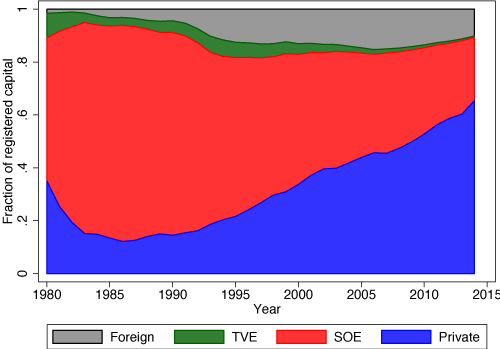
While China's growth has been impressive by any yardstick, what is perhaps most striking about this growth is that it occurred without the preconditions that are generally believed to be necessary for economic development, that is, without effective legal systems or well-functioning financial institutions (Allen et al. 2005). Whilst, the government did compensate for some of these institutional limitations by providing infrastructure and credit (Long and Zhang 2011, Wu 2016), it has been argued that informal mechanisms based on reputation and trust were probably the dynamics at play that allowed millions of entrepreneurs, most of whom were born in rural areas, to establish and grow their businesses (Peng 2004, Allen et al. 2005, Greif and Tabellini 2017). Moreover, case studies of production clusters such as Fleisher et al. (2010) and Nee and Opper (2012) indicate that long-established relationships among relatives and neighbours (from the same rural origin) substituted for legally enforced contracts between firms. Our analysis, which utilises comprehensive data covering the universe of registered firms over many years, advances this line of research by identifying and quantifying the role played by birth county networks in the growth of private enterprise in China.
Analytical approach
Our analysis focuses on variations of entry and capital investment of entrepreneurs with social connectedness of their birth county. We argue that (historical) population density is a good measure of social connectedness in a county. The reason is that the frequency of social interactions in counties is increasing in spatial proximity, which is proxied by population density. More frequent social interactions support higher levels of mutual help because news about deviations from cooperative behaviour travels more swiftly through the population, resulting in more effective social sanctions. This is based on the assumption that social heterogeneity within counties, which reduces cooperation, does not increase (too steeply) with population density. While this assumption may be plausible in rural counties, it is unlikely to be satisfied in cities that are typically composed of newcomers from a multitude of diverse communities. Consistent with these hypotheses, we show, using data from the China Family Panel Survey (CFPS) that the frequency of social interactions and the level of trust in local residents are significantly increasing with population density in counties but not in cities. The analysis therefore focuses on county-born entrepreneurs, whose firms constitute approximately two-thirds of all registered firms in China.
Theoretical model
We thereafter develop a theoretical model that describes how the dynamics of entry, concentration, and firm size vary with social connectedness. There are two roles for networks in the model: (i) sharing of knowhow, connections, and informal lending among entrepreneurs from a given origin that operate in a given destination; and (ii) access to information or referrals provided by incumbents from the same origin which channel new entrepreneurs into destinations occupied by the former.
These network effects reinforce each other, generating the following predictions:
- The flow of entrants from each origin is increasing over time, more steeply from more socially connected (higher population density) origins.
- Entrants are increasingly concentrated in particular sectors and locations over time, with this clustering increasing in origin social connectedness.
- Stronger networks allow less able individuals to enter business, resulting in a decline in initial firm size over time. This decline is steeper for entrants from more socially connected origins.
- Post-entry growth in firm size is determined by changes in the strength of the network. Thus, firms from more socially connected origins start smaller but subsequently grow faster.
Results
Each of these predictions is successfully tested with administrative data covering the universe of registered firms in China over the 1990-2009 period. These patterns in the data are evident in Figures 2 to 5.
Figure 2. Firm entry and population density
Figure 3. Sectoral concentration and population density
Figure 4. Marginal initial capital and population density
Figure 5. Asset growth and population density
We supplement the tests of the model’s predictions with a direct test of the mechanism underlying the network model. In particular, we estimate the relationship between initial entry and subsequent entry by firms from a given birth county in a particular sector-location. Consistent with a dynamic network multiplier effect, we find that one additional entrant from the birth county in the initial 1990-1994 period results in seven additional entrants over the 2000-2004 period and nine additional entrants over the 2005-2009 period. Moreover, the effects are stronger for firms from higher population density counties, and are concentrated within clans within counties. In contrast, conditional on the number of initial entrants from the birth county, the total number of initial entrants in the sector-location (aggregating across all origins) has no predictive power for the number of subsequent entrants. This striking result indicates that the birth county, or the clan within the birth county, is indeed the domain around which business networks are organised in China and that these networks operate independently within destinations.
Ruling out alternative explanations
While population density may be positively associated with social connectedness in a county, it could also be correlated with other characteristics that independently determine entry, concentration, or firm size. For example, population density is associated with education and the traditional occupational structure in a county, both of which could generate greater entry without requiring a role for networks. The empirical analysis addresses this concern by showing that the main predictions hold even after controlling for these variables. Moreover, these alternative factors would not explain the model’s predictions for concentration or firm size. Another possible concern is that higher population density origins could have better access to favourable destinations, with superior government infrastructure, or larger clusters of firms from whom new entrants could benefit. This hypothesis could account for many features of our model, but it would not explain why we observe greater entry, smaller initial firm size, and higher subsequent growth by firms from more socially connected origins within the same destination. In general, alternative explanations are consistent with some of our model’s predictions, but not all of them simultaneously.
Counterfactual experiment 1: What if networks were absent?
Having tested and validated the network-based model, we quantify the impact of these networks on firm entry and capital stocks. We estimate the structural parameters of the model and then conduct a counterfactual experiment in which the networks are shut down. Although the model is extremely parsimonious, it does a good job of matching entry and initial capital within sectors, across the range of birth county population densities, within the sample period for the structural estimation (1995-2004) and out of sample. The model estimates imply that in the absence of network effects, entry from county origins would have declined by as much as 64% over the 1995-2004 period (with a comparable decline in the total capital stock), which implies a 40% effect for the entire country.
Counterfactual experiment 2: What if entering firms received a credit subsidy?
We also examine another counterfactual policy experiment in which entering firms received a credit subsidy between 1995 and 2000. We show that a growth maximising strategy would target the subsidy to firms from higher population density counties which generate large network spillovers. With the targeted subsidy, entrants from those counties who are relatively small to begin with would become even smaller, thereby widening existing dispersion in firm size (across birth county networks). This dispersion arises despite the absence of mark-ups in output prices or wedges in factor prices, in contrast to much existing literature on firm misallocation in developing countries (Restuccia and Rogerson 2008, Hsieh and Klenow 2009). Hence, small firms and wide dispersion in firm size and productivity can be a consequence of efficiency-enhancing community-based networks that substitute for missing markets and formal institutions, rather than inefficient taxes or regulations. An additional implication of our analysis is that subsidies should account for intra-community spillovers over and above individual ability. However, the distributional consequences of growth-enhancing policies targeting more connected communities are complex; as such policies are likely to exacerbate inter-community inequality while promoting intra-community equity.
This article first appeared on VoxDev: https://voxdev.org/topic/firms-trade/community-networks-and-growth-private-enterprise-china
Further Reading
- Allen, Franklin, Jun Qian and Meijun Qian (2005), “Law, finance, and economic growth in China”, Journal of Financial Economics, 77(1): 57-116.
- Dai, Ruochen, Dilip Mookherjee, Kaivan Munshi and Xiabo Zhang (2018), ‘Community networks and the growth of private enterprise in China’, Working paper.
- Fleisher, Belton, Dinghuan Hu, William McGuire and Xiaobo Zhang (2010), “The evolution of an industrial cluster in China”, China Economic Review, 21(3): 456-469.
- Greif, Avner and Guido Tabellini (2017), “The clan and the corporation: Sustaining cooperation in China and Europe”, Journal of Comparative Economics, 45(1): 1-35. Available here.
- Hsieh, Chang-Tai and Peter J Klenow (2009), “Misallocation and manufacturing TFP in China and India”, Quarterly Journal of Economics, 124(4): 1403-1448.
- Long, Cheryl and Xiaobo Zhang (2011), “Cluster-based industrialization in China: Financing and performance”, Journal of International Economics, 84(1): 112-123.
- Long, Cheryl and Xiaobo Zhang (2012), “Patterns of China's industrialization: Concentration, specialization, and clustering”, China Economic Review, 23(3): 593-612.
- Nee, V and S Opper (2012), Capitalism from Below: Markets and Institutional Change in China, Harvard University Press.
- Peng, Yusheng (2004), “Kinship networks and entrepreneurs in China's transitional economy”, American Journal of Sociology, 109(5): 1045-1074.
- Restuccia, Diego and Richard Rogerson (2008), “Policy distortions and aggregate productivity with heterogeneous establishments”, Review of Economic Dynamics, 11(4): 707-720.
- Wu, Mark (2016), “The “China, Inc.” Challenge to Global Trade Governance”, Harvard International Law Journal, 57(2): 261-324.
Comments will be held for moderation. Your contact information will not be made public.