It is well known that managerial quality impacts firm productivity and growth. Which managerial skills, traits, and practices contribute most to productivity? Are all such traits easily observable? Are such traits appropriately rewarded in pay? Based on two years of daily, line-level production data from a large Indian garment firm and rich survey data on line managers, this article seeks to address these questions.
Studies show that managerial quality plays a significant role in determining firm productivity and growth, thus in part contributing to productivity gaps across rich and poor countries (Caselli 2005, Hall and Jones 1999). If firm productivity is indeed a function of management, firms that overlook investing in improving their managerial quality may suffer a competitive disadvantage in the longer run. But what skills, traits, and practices constitute high-quality management? Identifying good managers, both among candidates at the time of hiring and among employees at the time of promotion, for the purposes of rewarding best practices and targeting training in deficient skills, is difficult and costly, particularly for low-margin, labour-intensive manufacturing firms in developing countries.
The need of the hour then shifts to answering some basic questions: which managerial skills, traits, and practices contribute most to productivity? Are all such traits easily observable? Are such traits appropriately rewarded in pay? A study conducted by Good Business Lab1 in garment factories seeks to address these exact questions, leveraging granular production data and rich measures of managerial quality across many production lines.
Setting context
The garment industry, which is concentrated in low-income countries, remains highly labour intensive. There are three broad stages of garment production: cutting, sewing, and finishing. In this study, we focus on the sewing department, wherein garments are sewn in production lines consisting of around 50-70 workers arranged in groups of machine operations, one worker to each sewing machine. Lines are managed by production supervisors or managers. The sewing department forms the majority of the workforce in the garment industry, lends itself to measurement of individual productivity and task complexity, thus making it the focus of our study.
We use two years of granular production data, from July 2013 to June 2015, of six garment factories operated by Shahi Exports, Pvt. Ltd., in Bengaluru, India. Firstly, we obtained rich data on daily productivity of production-line workers and information on specific garment styles being produced by each line every day. Secondly, to measure managerial quality, we conducted a survey of all production-line managers that reported a wide array of features, some of which were easily observable, like industry experience, while others more costly and difficult to ascertain at the time of hiring, like personality psychometrics or managerial attention. We match these two datasets for each manager to answer the basic question: which managerial skills, traits, and practices contribute most to productivity? After carefully estimating the complicated relationship between managerial quality and productivity, we go a step further to find out the impacts of screening/hiring policies and management training interventions on productivity by data simulation. In addition to mapping the managerial attributes which impact productivity, we also investigate how these attributes are priced into wages; specifically, whether the attributes that matter most for productivity are commensurately weighted for in managers’ wages.
Productivity and its drivers
We begin by defining productivity and understanding what drives it.
Learning and forgetting (on the part of workers)
Efficiency (the industry standard measure of productivity) is defined as the proportion of targeted production achieved per unit time. One key concept that helps us understand and model productivity in this context is the learning curve. Learning by doing is a huge driver of economic growth across many sectors, but it is a particularly foundational feature of labour-intensive manufacturing. The more experience workers accumulate doing the specific tasks they are responsible for, the faster they will be able to do them (and the less mistakes they will make).
Figure 1 shows the basic learning curve in the garment production process. At the beginning of new ‘styles’, for example a white t-shirt, production lines start at a low efficiency level, around 40%. But after about two weeks of making the same style they converge to a ‘peak’ efficiency of around 60%. This is due to learning by doing.
Figure 1. Efficiency by days running

Along with learning comes forgetting – or what economists call a depreciation of knowledge over time – which is also an important driver of productivity. Figure 2 depicts how production lines ‘forget’ how to make styles efficiently if they have not made them for more than a few weeks.
Figure 2. Forgetting (prior days)

Key managerial characteristics
Keeping the aforementioned frame in mind, we focus on seven dimensions of managerial characteristics: tenure, demographics, cognitive skills, control, personality, autonomy, and attention. We use detailed surveys of production line managers to quantify what behaviours and traits are really useful in changing different parts of this learning process. The rich managerial-quality data, like the line-productivity data at the daily level, enable us to study the ways in which these managerial attributes interact with the learning-by-doing process. We find that learning curves are significantly different for managers with differing ‘quality’.
First, we look at easy-to-screen dimensions such as tenure in a supervisory position (Figure 3). We find that managers who have been supervising the current production line for longer enable their lines to start an order at higher levels of efficiency and increase their productivity faster compared to less tenured managers.
Figure 3. Tenure supervising current line

We also look at more difficult- and costly-to-screen personality characteristics and managerial practices that might also be important for enabling line productivity. Managers that have a more internal locus of control (that is, stronger beliefs that they can control things as opposed to being impacted mostly by luck or fate) enable their lines to achieve faster learned productivity as well (Figure 4a).
Figure 4a. Internal locus of control

Lines supervised by more psychologically distressed managers start at lower levels of initial productivity (Figure 4b).
Figure 4b. Psychological distress

We also find that lines supervised by more autonomous (more autonomous problem-solving) and attentive (more frequent monitoring) supervisors enable faster learning-by-doing across the life cycle of a production run (Figures 5a and 5b).
Figure 5a. Autonomous problem-solving
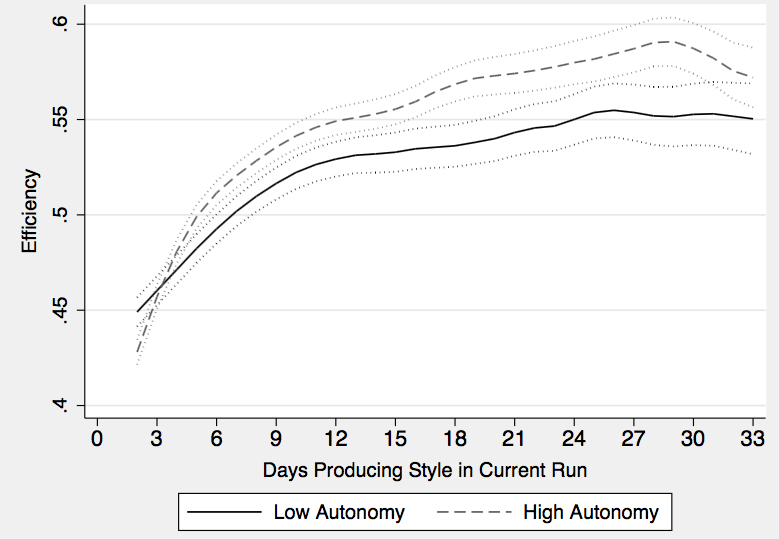
Figure 5b. Monitoring frequency

Similarly, managers with higher cognitive skills, such as better memory for numbers (like how many shirt-segments each worker made over the last order) enable lines to start new orders at higher initial levels of productivity (Figure 6).
Figure 6. Digit span recall

Simulations of screening and training policies
Armed with these complex interrelationships, we simulate the contribution of an increase in each of the factors that can be screened at the time of hiring and each of the factors corresponding to behaviours and practices in which supervisors can be trained. Of course, the decisions of which policy – screening or training – and which dimensions to prioritise depend also on corresponding impacts on the wages needed to retain these higher-quality supervisors.
Because we also have data on how much managers with different characteristics and achieving different levels of productivity are being paid, we are also able to simulate what each simulated manager would be paid. Combined with the productivity simulations, this is a powerful tool to understand whether the firm can improve its stock of managerial quality, either by training existing managers or selecting better managers in the hiring process, and how much these improvements would likely cost.
Perhaps surprisingly, many important dimensions of managerial quality are not being priced into manager pay appropriately. The traits and practices that are least rewarded tend to be the ones that are hardest to measure or observe at the time of hiring. This is consistent with the employer (and competing potential employers) not knowing which traits and practices are most productive and whether a particular manager exhibits those dimensions of quality.
Taking learnings forward
Our results imply a significant opportunity. If firms can figure out how to cheaply measure some difficult- and costly-to-observe but productive traits (like a limited belief in luck or fate) and train managers in under-emphasised but productive practices (like attention), they can enable lines to start orders at higher levels of productivity, learn how to be more productive faster, and retain the learned productivity longer. Armed with these implications, we are developing a low-cost, scalable technology on the ground to enable screening and subsequently training managers in precisely these qualities.
Notes:
- Good Business Lab (GBL) is a labour innovation lab that designs, rigorously tests, and scales up interventions, which aim to deliver both improvements in worker well-being and business returns. Achyuta Adhvaryu and Anant Nyshadham are two of the co-founders of GBL.
Further Reading
- Adhvaryu, A, A Nyshadham and JA Tamayo (2019), ‘Managerial Quality and Productivity Dynamics’, National Bureau of Economic Research (NBER) Working Paper No. 25852, May 2019.
- Caselli, Francesco (2005), “Accounting for cross-country income differences”, Handbook of Economic Growth, 1:679-741
- Hall, Robert E and Charles I Jones (1999), “Why do some countries produce so much more output per worker than others?”, The Quarterly Journal of Economics, 114(1):83-116.
Comments will be held for moderation. Your contact information will not be made public.